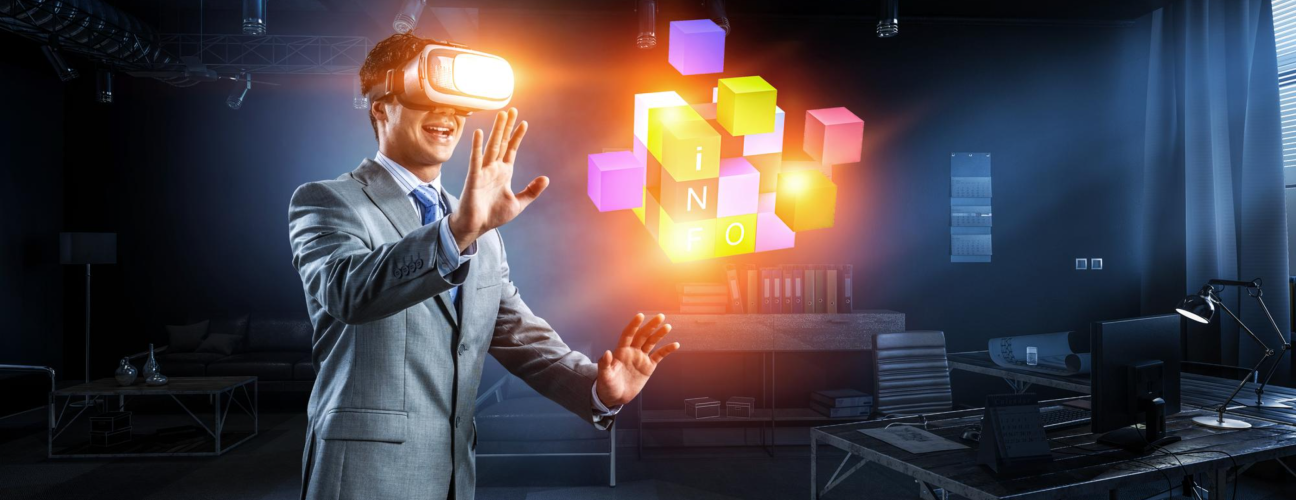
Unveiling the Black Box: Exploring Explainable AI in Healthcare
In recent years, artificial intelligence (AI) has emerged as a transformative force in the field of healthcare, promising unprecedented insights and efficiency. From disease diagnosis to treatment optimization, AI systems are increasingly being integrated into various facets of medical practice. However, as AI algorithms become more complex and sophisticated, they often operate as “black boxes,” making it challenging for healthcare professionals and patients to understand the rationale behind their decisions. This lack of transparency not only raises concerns about the reliability and trustworthiness of AI-driven solutions but also poses significant ethical and regulatory challenges.
Bridging the Gap between Complexity and Transparency
Enter explainable AI (XAI) – an emerging field that seeks to demystify the decision-making processes of AI systems and enhance their transparency. By providing interpretable explanations for AI-generated outcomes, XAI holds the potential to instill confidence in these systems and foster greater trust among stakeholders. In the context of healthcare, the importance of explainability cannot be overstated, as the consequences of AI errors or biases can have profound implications for patient safety and well-being.
So, what exactly is explainable AI, and how can it benefit the healthcare industry?
At its core, explainable AI refers to the ability of AI systems to provide human-understandable explanations for their predictions or decisions. This entails not only revealing the factors or features that influenced a particular outcome but also elucidating the underlying reasoning or logic behind the decision-making process. In essence, explainable AI aims to bridge the gap between the “black box” nature of AI algorithms and the need for transparency and accountability in decision-making.
In the realm of healthcare, explainable AI offers several compelling advantages:
- Enhanced Trust and Acceptance: By shedding light on the inner workings of AI systems, explainable AI can help build trust and acceptance among healthcare professionals, patients, and regulatory authorities. When clinicians understand why a certain diagnosis or treatment recommendation was made by an AI algorithm, they are more likely to trust its judgment and incorporate it into their decision-making process.
- Insight into Clinical Decision-Making: Explainable AI can provide valuable insights into the complex interplay of variables and features that influence clinical outcomes. By elucidating the factors driving AI predictions, clinicians can gain a deeper understanding of disease mechanisms, patient risk profiles, and treatment responses. This, in turn, can inform more personalized and effective patient care strategies.
- Detection of Bias and Errors: Transparent AI models enable the identification and mitigation of bias and errors that may creep into the decision-making process. By examining the rationale behind AI predictions, clinicians can detect and rectify instances of algorithmic bias, ensuring fair and equitable treatment for all patients.
- Compliance with Regulatory Standards: In an increasingly regulated healthcare landscape, explainable AI can help organizations demonstrate compliance with regulatory standards and guidelines. By providing interpretable explanations for AI-driven decisions, healthcare providers can satisfy the transparency and accountability requirements set forth by regulatory bodies.
Despite its potential benefits, implementing explainable AI in healthcare poses several challenges. Achieving transparency without sacrificing predictive performance, navigating the trade-off between simplicity and accuracy in explanation models, and ensuring the security and privacy of sensitive patient data are just a few of the complex issues that must be addressed.
Nevertheless, the growing interest and investment in explainable AI reflect its importance as a foundational element of trustworthy and ethical AI systems. As healthcare organizations continue to leverage AI technologies to improve patient care and outcomes, prioritizing explainability will be essential for fostering trust, mitigating risks, and realizing the full potential of AI in healthcare.
Conclusion
In conclusion, the journey towards unlocking the potential of AI in healthcare begins with unveiling the black box and embracing the principles of explainable AI. By fostering transparency, accountability, and trust, explainable AI can empower clinicians, engage patients, and revolutionize the delivery of healthcare in the 21st century.